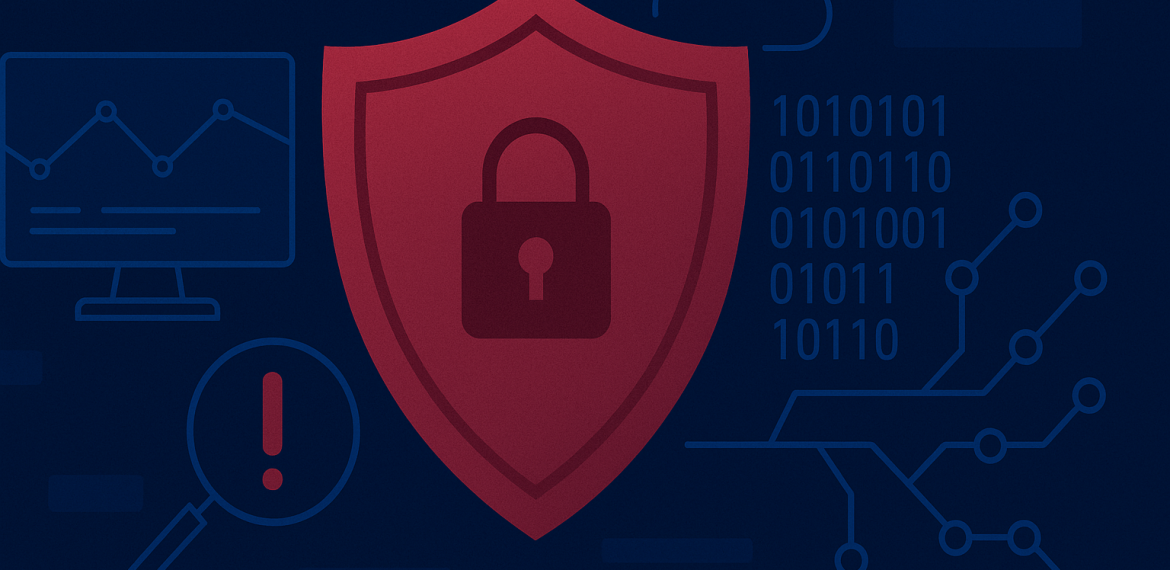
Using AI to Detect Anomalies Before They Become Breaches
Using AI to Detect Anomalies Before They Become Breaches
Why Early Anomaly Detection Is Crucial
In the modern cybersecurity landscape, the difference between a near-miss and a major breach often boils down to one thing: timing. Cyberattacks rarely appear out of nowhere — they usually begin with subtle anomalies that hint at deeper, malicious activity. But traditional security systems often miss these early warning signs, overwhelmed by noise or reliant on static rules. That’s where Artificial Intelligence (AI) comes in.
AI excels at spotting the unusual — the login at an odd hour, the slight deviation in user behavior, the unexpected spike in network traffic. With AI-driven anomaly detection, enterprises can spot threats long before they escalate into full-scale breaches. In this blog, we explore how AI is changing the game in threat detection by identifying anomalies early, accurately, and at scale.
Understanding Anomalies: What Are We Really Looking For?
Anomalies are deviations from normal behavior — patterns that don’t conform to established baselines. In cybersecurity, anomalies can include:
- Login attempts from unusual locations or devices
- Sudden spikes in data access or file downloads
- Unfamiliar process executions or command-line usage
- Unexpected behavior from privileged accounts
- Communication with suspicious external domains
While some anomalies are harmless, others may be the first signs of compromise. The challenge lies in distinguishing between benign and malicious anomalies quickly and accurately — something AI is uniquely suited to do.
How AI Detects Anomalies in Real Time
Unlike rule-based systems that require predefined thresholds or conditions, AI leverages machine learning to learn what “normal” looks like — and continuously adapts that definition over time. Here’s how:
- Behavioral Baselines: AI models establish baseline behavior for users, devices, and applications by analyzing historical data.
- Unsupervised Learning: Without needing labeled data, unsupervised models detect outliers in patterns, flagging anything that deviates from the learned baseline.
- Real-Time Analysis: AI systems can ingest and analyze massive volumes of logs, metrics, and events in real time, detecting anomalies within seconds.
- Correlation Across Sources: AI correlates signals from multiple sources — endpoints, servers, cloud workloads — to build a more accurate threat picture.
This multi-dimensional, adaptive approach gives AI an edge over traditional tools when it comes to catching threats early.
Reducing False Positives Through AI Contextualization
One of the biggest challenges in anomaly detection is the flood of false positives. Not every unusual action is a threat — and constant alerts can lead to alert fatigue and missed real threats.
AI helps address this in several ways:
- Contextual Awareness: AI considers contextual factors — user roles, access history, device health, time of day — to assess whether an anomaly is suspicious.
- Risk Scoring: AI assigns a risk score to each anomaly, helping prioritize the most urgent and credible threats.
- Pattern Recognition: By analyzing broader patterns over time, AI can determine whether an isolated anomaly is part of a coordinated attack.
This refinement dramatically reduces noise, allowing security teams to focus on genuine threats.
AI in Action: Real-World Use Cases of Anomaly Detection
AI-powered anomaly detection is already delivering results in enterprise environments. Some common use cases include:
- Insider Threat Detection: AI can identify subtle behavioral changes — such as accessing sensitive files or working outside normal hours — that may indicate insider risk.
- Credential Theft and Account Takeover: If a user’s login behavior suddenly changes (new device, location, access pattern), AI can detect and flag the session.
- Ransomware Precursors: AI can detect unusual encryption patterns, lateral movement, and privilege escalation before ransomware executes.
- Phishing and Business Email Compromise (BEC): AI analyzes communication patterns to identify suspicious emails and impersonation attempts.
- Cloud Misuse: AI identifies unusual API calls, resource provisioning, or cross-region data transfers that could signal misconfigurations or attacks.
These use cases show how early anomaly detection can make the difference between containment and catastrophe.
Integration with Security Tools and Platforms
AI-based anomaly detection is most powerful when integrated into the broader security ecosystem. It feeds intelligence into other tools and workflows, including:
- Security Information and Event Management (SIEM): Enhances correlation, threat scoring, and alerting within SIEM platforms.
- Security Orchestration, Automation, and Response (SOAR): Triggers automated responses based on anomaly risk level — like isolating devices or disabling accounts.
- Endpoint Detection and Response (EDR): Combines endpoint telemetry with anomaly detection to spot and respond to suspicious behavior locally.
- User and Entity Behavior Analytics (UEBA): Focuses specifically on user-driven anomalies, enabling identity-centric security strategies.
This interconnected approach creates a layered defense mechanism that adapts and evolves with your organization’s needs.
Benefits of Using AI for Proactive Breach Prevention
The shift from reactive to proactive cybersecurity brings numerous benefits:
- Faster Threat Detection: AI spots threats in real time, reducing dwell time and minimizing potential damage.
- Improved Accuracy: Machine learning reduces false positives and sharpens precision over time.
- Scalability: AI handles massive datasets across hybrid environments — on-premises, cloud, and endpoints — without performance degradation.
- Cost Savings: Early detection prevents breaches, saving organizations millions in potential losses, remediation costs, and reputational damage.
- Empowered Security Teams: AI augments human analysts with better tools and insights, allowing them to act more strategically.
By leveraging AI, organizations can shift their focus from firefighting to forward planning.
Overcoming Challenges in AI-Based Anomaly Detection
While the advantages are significant, implementing AI for anomaly detection comes with challenges:
- Data Quality and Diversity: Poor-quality or incomplete data reduces AI accuracy. Diverse data sources must be normalized and unified.
- Model Drift: As environments evolve, AI models must be continuously trained to stay relevant.
- Explainability: Security teams need to understand why an anomaly was flagged. Explainable AI (XAI) is key to trust and adoption.
- Privacy and Compliance: Behavioral monitoring must comply with data protection regulations and internal privacy policies.
- Human Oversight: AI is not infallible. Human-in-the-loop validation ensures the right balance of automation and accountability.
With the right strategy and governance, these challenges can be effectively managed.
How Buxton Can Help
At Buxton Consulting, we help organizations harness the full potential of AI to detect anomalies early and stop breaches before they happen. Our team brings deep expertise in cybersecurity, data science, and enterprise AI deployment.
Our AI-powered security services include:
- Advanced Anomaly Detection Solutions: Custom machine learning models tailored to your environment, data, and risk profile.
- End-to-End Integration: Seamless deployment into your existing SIEM, SOAR, and EDR platforms.
- Behavioral Analytics and UEBA: Insightful monitoring of user and entity behavior with dynamic risk scoring.
- Threat Intelligence Augmentation: Combining anomaly detection with threat intel for enhanced context.
- Real-Time Monitoring and Response: 24/7 managed detection services with AI-enabled alerting and response workflows.
Why partner with Buxton?
- Proven track record in AI and enterprise security
- Industry-leading tools and frameworks
- Rapid deployment and continuous tuning
- Dedicated support and compliance alignment
Buxton empowers you to be proactive, not reactive — turning every anomaly into an opportunity to strengthen your defenses.
Conclusion: Don’t Wait for the Breach
In today’s threat landscape, waiting for alerts from traditional systems is no longer enough. Breaches don’t start with fireworks — they start with whispers. And AI gives you the ears to hear them.
By adopting AI-powered anomaly detection, organizations can uncover hidden threats, reduce risk, and stay one step ahead of cyber adversaries. It’s not just about catching attacks — it’s about preventing them before they begin.
Now is the time to make AI an integral part of your cybersecurity strategy. Because when it comes to breaches, early detection isn’t just helpful — it’s everything.