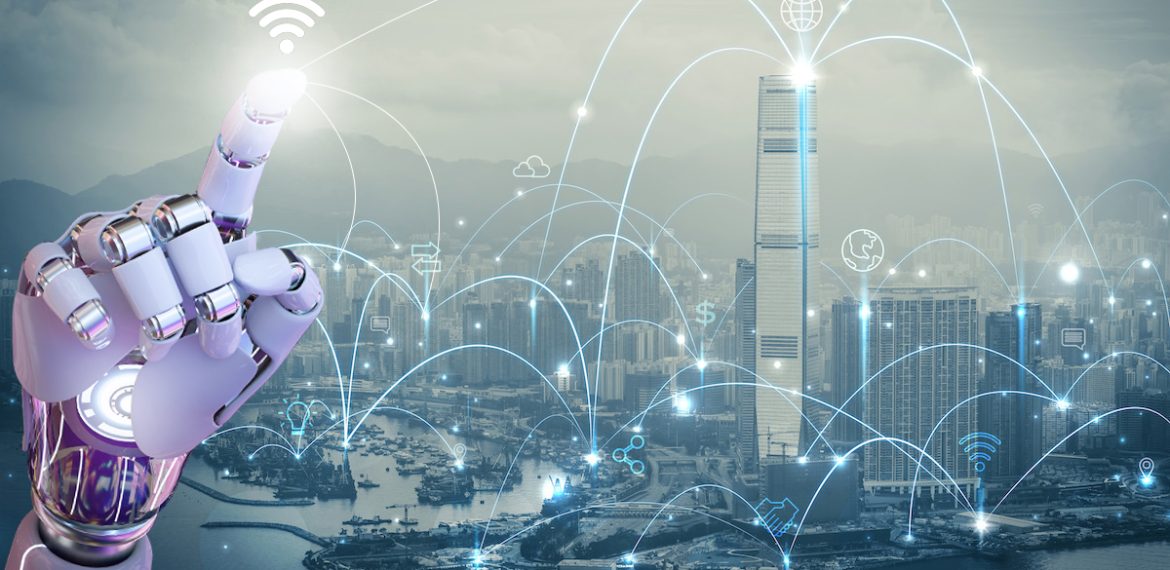
Predictive Network Maintenance with AI: Stay Ahead of Failures
Predictive Network Maintenance with AI: Stay Ahead of Failures
A single unexpected network failure can ripple through an organization, disrupting operations, degrading customer experience, and driving up costs. Traditional maintenance models, which rely on scheduled inspections or reacting to failures after they occur, are no longer sufficient to meet the demands of modern digital infrastructure.
As enterprise networks grow more complex—spanning data centers, cloud platforms, edge devices, and global branches—the ability to predict and prevent failures before they happen has become a game-changer. Enter predictive network maintenance, powered by artificial intelligence.
By leveraging real-time data, machine learning, and intelligent automation, organizations can now move from a reactive stance to a proactive one—identifying potential issues early, optimizing performance, and dramatically reducing downtime. This blog explores how AI is redefining network maintenance, what makes predictive strategies effective, and how your organization can harness these technologies to stay ahead of network disruptions.
The Problem with Traditional Network Maintenance
For decades, organizations have relied on reactive or time-based network maintenance—waiting for something to break, or performing routine checks based on fixed schedules. While these methods offer a degree of control, they often fall short in today’s fast-paced, hyperconnected environments.
Key issues with traditional approaches:
- Reactive firefighting: Teams are often blindsided by sudden failures, forcing a scramble to diagnose and resolve issues under pressure.
- Wasted resources: Scheduled maintenance can lead to unnecessary downtime or part replacements, even when systems are operating normally.
- Limited visibility: Conventional monitoring tools may only flag problems once they’ve already impacted performance, leaving little time to mitigate damage.
- Data overload without insights: Even with logs and alerts, IT teams struggle to interpret signals in real time, making it difficult to spot patterns or early warning signs.
In an age where every minute of downtime can cost thousands—or even millions—of dollars, organizations need smarter solutions. That’s where predictive maintenance comes into play: using AI to anticipate failures before they happen, enabling faster response and better outcomes.
What is Predictive Network Maintenance?
Predictive Network Maintenance is a proactive approach that leverages data analytics, artificial intelligence (AI), and machine learning (ML) to foresee potential network issues before they disrupt operations. Instead of reacting to failures or relying solely on scheduled maintenance, this method analyzes patterns in network performance data to predict when and where problems are likely to occur.
At its core, predictive maintenance answers questions like:
- Which devices are likely to fail in the next week?
- Are there performance trends indicating a deeper issue?
- What’s the likelihood of a bandwidth bottleneck during peak hours?
How it works:
- Data Collection: Continuous monitoring of network devices, traffic patterns, logs, and performance metrics.
- Pattern Recognition: AI algorithms analyze historical and real-time data to identify signs of wear, anomalies, or degradation.
- Failure Prediction: Machine learning models forecast issues like hardware failure, packet loss, latency spikes, or service degradation.
- Actionable Alerts: The system triggers alerts or automated responses—sometimes even before users notice a slowdown.
Key Difference:
- Preventive Maintenance is scheduled and generic—“check every router monthly.”
- Predictive Maintenance is dynamic and data-driven—“this router is showing abnormal packet drop trends and may fail in 3 days.”
This approach shifts the IT team’s role from fixing problems to avoiding them—improving uptime, efficiency, and peace of mind.
Role of AI in Predictive Network Maintenance
Artificial Intelligence is the engine that powers predictive network maintenance. It enables IT teams to move beyond static monitoring dashboards and embrace intelligent systems that learn, adapt, and act. AI doesn’t just highlight symptoms—it uncovers root causes, anticipates failures, and often recommends or executes corrective actions.
Here’s how AI drives predictive maintenance:
1. AI-Powered Monitoring
Traditional monitoring tools rely on fixed thresholds and manual interpretation. AI changes the game by continuously learning from your network’s behavior. It dynamically adjusts baselines, detects subtle shifts, and flags deviations that humans or static systems might overlook.
2. Machine Learning for Failure Prediction
ML models are trained on historical data—such as device logs, incident tickets, and performance metrics—to identify failure patterns. Over time, these models become better at predicting conditions that lead to hardware failures, link congestion, or performance degradation.
3. Anomaly Detection at Scale
AI algorithms scan thousands of data points across routers, switches, servers, and cloud interfaces in real-time. They detect anomalies such as unusual traffic spikes, abnormal CPU/memory usage, or latency variations that could signal an impending issue.
4. Natural Language Processing (NLP)
NLP can parse unstructured data—like support tickets, technician notes, or system logs—to uncover trends and hidden issues. This allows the system to “read between the lines” and understand contextual warnings before they escalate.
5. Smart Alerts and Automated Response
AI systems can generate prioritized alerts with contextual insights, reducing alert fatigue and helping teams focus on what matters most. In advanced setups, AI even triggers automated remediation—rerouting traffic, restarting services, or opening support tickets automatically.
Benefits of AI-Driven Predictive Maintenance
Implementing AI in your network maintenance strategy isn’t just a technical upgrade—it’s a strategic advantage. By anticipating issues before they become incidents, AI-powered predictive maintenance delivers tangible business value across the board.
1. Minimized Downtime
Predictive models identify potential failure points early, allowing IT teams to take action before outages occur. This reduces unplanned downtime, service disruptions, and their associated costs.
2. Cost Efficiency
Avoiding emergency fixes, unnecessary equipment replacements, and SLA penalties leads to significant savings. Predictive maintenance also enables more efficient resource allocation, both human and hardware.
3. Enhanced Network Performance
AI helps maintain optimal performance by flagging degradations—such as bandwidth congestion or hardware fatigue—before they affect end users. The result: faster, smoother, more reliable digital experiences.
4. Improved User Satisfaction
When networks run seamlessly, customers, employees, and partners benefit from uninterrupted access to services and applications. Fewer disruptions lead to higher satisfaction and trust.
5. Smarter Resource Planning
Predictive insights allow IT teams to schedule maintenance activities more strategically, avoiding business-critical windows and reducing reliance on firefighting.
6. Data-Driven Decision Making
AI provides actionable insights—not just alerts. IT leaders can use these analytics to prioritize investments, justify upgrades, and refine infrastructure strategy.
7. Scalability and Automation
As networks expand in complexity and size, manual monitoring becomes unsustainable. AI scales effortlessly, learning across devices, environments, and locations—while automating tasks that once required human intervention.
Core Components of a Predictive Maintenance Framework
To successfully implement AI-driven predictive network maintenance, organizations need a well-structured framework that brings together data, technology, and process integration. It’s not just about deploying a tool—it’s about creating a smart, responsive system built to evolve.
1. Data Collection & Integration
- The foundation of predictive maintenance lies in comprehensive data collection.
- Sources include: routers, switches, firewalls, SD-WAN devices, application logs, cloud services, and user traffic.
- This data must be centralized and normalized for effective analysis—often through APIs, telemetry protocols (like SNMP or NetFlow), and log aggregators.
2. Anomaly Detection Engines
- AI and ML models continuously analyze incoming data streams to detect deviations from normal behavior.
- These engines identify unusual patterns such as packet loss, latency spikes, hardware temperature rises, or memory usage anomalies.
- Over time, they adapt and improve as they’re exposed to more network behavior.
3. Predictive Analytics Dashboard
- A centralized interface for IT teams to visualize health metrics, forecast risks, and take action.
- Dashboards present KPIs like device health scores, predicted failure timelines, and confidence levels of each prediction.
- They also offer drill-down capabilities to investigate specific devices, incidents, or trends.
4. Automated Workflows & Integrations
- The framework should support integrations with ITSM platforms (e.g., ServiceNow, Jira), NOC tools, or orchestration layers.
- This allows the system to automatically open tickets, trigger remediation scripts, or escalate high-priority alerts.
- Automation reduces response time and ensures consistency in how issues are addressed.
5. Feedback Loop & Model Training
- Continuous improvement is key—feedback from resolved incidents and technician actions is fed back into the AI models.
- This refines prediction accuracy, reduces false positives, and adapts to changing network environments.
Real-World Use Cases (Hypothetical)
Predictive network maintenance powered by AI isn’t just theory—it’s being implemented across industries to drive measurable improvements in reliability, cost savings, and user experience. Here are a few real-world hypothetical scenarios where predictive maintenance can deliver an impact-
1. Telecom Providers Can Reduce Outages Across Rural Networks
A regional telecom company is struggling with frequent hardware failures in remote cell towers. By deploying AI-driven monitoring and predictive analytics, they can:
- Detect early warning signs of signal degradation and battery failure.
- Automatically dispatch field technicians with the right parts before customers are affected.
- Reduce unscheduled outages by 42% and cut mean time to repair (MTTR) by half.
2. Enterprise IT Team Can Avoid SLA Penalties
A global enterprise managing thousands of networked devices across offices and cloud platforms can use predictive models to analyze log data, device health, and traffic behavior.
- Identification of 80% of high-risk routers before failures occurred.
- Avoided multiple SLA violations with early alerts and proactive fixes.
- Enhanced visibility for their NOC team via an AI-powered dashboard.
3. Cloud Service Provider Can Optimize Hardware Refresh Cycles
Rather than replacing servers on fixed schedules, a cloud provider can leverage predictive maintenance to:
- Use machine learning to track CPU load, fan speed trends, and thermal data.
- Predict when server components were likely to fail based on historical patterns.
- Extend hardware life by 18% while reducing emergency replacements.
4. Smart City Infrastructure Stays Resilient
In a smart city project, predictive maintenance can help city authorities monitor connected traffic systems, public Wi-Fi access points, and surveillance networks. Benefits included:
- Timely identification of failing nodes in surveillance systems.
- Prevention of critical outages in high-traffic zones through automated network rerouting.
- Increased operational uptime of urban infrastructure.
Challenges and Considerations
While the benefits of AI-driven predictive network maintenance are compelling, successful implementation requires navigating a few critical challenges. Understanding these upfront can help organizations set realistic expectations and plan for a smoother deployment.
- Data Quality and Availability
- Garbage in, garbage out: Predictive models are only as good as the data they learn from.
- Incomplete logs, inconsistent formatting, or missing telemetry data can compromise model accuracy.
- Organizations must invest in clean, structured, and comprehensive data collection across all network components.
- False Positives and Negatives
- AI models may occasionally trigger false alarms or miss genuine issues, especially in early stages.
- This can lead to alert fatigue or distrust in the system.
- Ongoing model tuning, feedback loops, and human-in-the-loop oversight are essential to improve reliability.
- Integration with Legacy Systems
- Many enterprises still operate a mix of legacy hardware, custom applications, and outdated monitoring tools.
- These components may lack APIs or standard telemetry protocols, making integration more complex.
- A phased or hybrid approach is often required to bridge old and new infrastructure.
- Skills Gap
- Deploying and managing AI models requires specialized skills in data science, network engineering, and automation.
- Internal teams may need training, or organizations may choose to partner with external service providers.
- Security and Compliance
- Real-time network monitoring and data aggregation raise concerns around data privacy and regulatory compliance.
- Organizations must ensure that predictive systems adhere to frameworks like GDPR, HIPAA, or regional cybersecurity standards.
- Upfront Investment
- Building a predictive framework demands investment in AI platforms, sensors, storage, and analytics infrastructure.
- However, many companies recover this investment quickly through reduced downtime, fewer manual interventions, and improved customer satisfaction.
How Buxton Can Help: Smarter Network Management with AI
At Buxton, we understand that keeping your network running smoothly is mission-critical—but doing it manually in today’s complex IT environment is no longer sustainable. That’s why we offer Managed Network Management Services designed to help you stay ahead of failures using the power of AI and automation.
Our Approach: Proactive, Predictive, and Intelligent
We go beyond traditional monitoring to deliver a data-driven, AI-enhanced network management solution that gives you complete visibility and control—without the operational burden.
Here’s how we support your network’s health and performance:
✅ AI-Powered Monitoring & Alerts
- Real-time analysis of network traffic, device performance, and environmental factors.
- Early detection of anomalies and potential failure points—before they disrupt operations.
✅ Predictive Analytics & Health Scoring
- Machine learning models forecast issues such as hardware degradation, bandwidth spikes, or service bottlenecks.
- Custom health scores and failure likelihood indicators help prioritize preventive actions.
✅ Integrated Incident Management
- Smart workflows that trigger automated responses or generate ITSM tickets.
- Seamless integration with your existing tools like ServiceNow, Jira, or custom NOC dashboards.
✅ 24/7 Expert Oversight
- Our certified network engineers monitor and manage your infrastructure around the clock.
- Rapid response and root cause analysis ensure minimal downtime and quick recovery.
✅ Scalable and Customizable
- Whether you manage a few branches or a global hybrid environment, our solution scales with you.
- Tailored SLAs, compliance-ready architecture, and industry-specific support.
Conclusion
As networks grow in complexity and business expectations rise, the old ways of maintaining infrastructure simply can’t keep up. Predictive network maintenance—powered by AI—is no longer a luxury; it’s a necessity for organizations that value uptime, agility, and operational efficiency.
By anticipating issues before they escalate into failures, businesses can not only reduce downtime and costs but also deliver consistently excellent digital experiences. With the right data, tools, and expertise, predictive maintenance becomes a powerful enabler of business continuity and growth.
AI doesn’t just help you respond faster—it helps you stay ahead.
At Buxton, we bring together advanced AI models, proven network management strategies, and 24/7 expert support to help you take control of your network’s future.
👉 Contact us today to schedule a free assessment and see how our Managed Network Management Services can keep you steps ahead of failures.
Stay proactive. Stay resilient. Stay ahead—with Buxton.